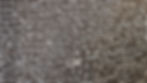
Quant
Quantitative investing is a strategy that uses data-driven, systematic approaches to make investment decisions. It relies on advanced statistical and machine learning techniques to analyze large datasets and identify patterns that can be used to predict future market movements. Data science plays a key role in quantitative investing, providing the tools and techniques needed to extract insights and knowledge from data.
The history of quantitative investing dates back to the 1960s, when financial economists such as Harry Markowitz and William Sharpe developed the concept of modern portfolio theory, which introduced the use of statistical analysis to optimize investment portfolios. In the 1980s and 1990s, the development of computers and statistical software made it possible to analyze larger and more complex datasets, leading to the growth of quantitative investment strategies such as trend following and statistical arbitrage.
Quantitative investing has had a number of major successes, including the development of the first exchange-traded fund (ETF) by State Street Global Advisors in 1993, which revolutionized the way investors access diverse portfolios. In the early 2000s, quantitative hedge funds such as Renaissance Technologies and DE Shaw gained widespread attention for their impressive returns and innovative approaches to investment.
Today, quantitative investing is a well-established strategy used by a wide range of investors, including hedge funds, asset managers, and individual investors. It is particularly popular in the financial industry, where data science and machine learning techniques are used to analyze market trends and identify trading opportunities.
Below are 10 most popular quantitative trading strategies in the industry
High-frequency trading: High-frequency trading is a quantitative investment strategy that involves making rapid trades based on algorithms that analyze large amounts of data in real-time.
Trend following: Trend following is a quantitative investment strategy that involves identifying trends in the market and buying or selling based on those trends.
Statistical arbitrage: Statistical arbitrage is a quantitative investment strategy that involves identifying statistical discrepancies in the market and making trades to profit from those discrepancies.
Risk parity: Risk parity is a quantitative investment strategy that involves allocating capital across different asset classes in order to achieve a balanced level of risk.
Smart beta: Smart beta is a quantitative investment strategy that involves using alternative weighting schemes to construct portfolios, such as weighting stocks by fundamental metrics rather than market capitalization.
Factor investing: Factor investing is a quantitative investment strategy that involves identifying and investing in factors that have been shown to predict returns, such as value, momentum, and size.
Volatility arbitrage: Volatility arbitrage is a quantitative investment strategy that involves buying and selling options to profit from differences in implied and realized volatility.
Systematic global macro: Systematic global macro is a quantitative investment strategy that involves using algorithms to analyze and trade on global macroeconomic trends.
Quantitative easing: Quantitative easing is a monetary policy tool used by central banks to stimulate the economy by purchasing government bonds and other securities. It can also be used as a quantitative investment strategy by investors who seek to profit from changes in monetary policy.
Quantitative asset allocation: data-driven investment strategy that uses algorithms and statistical analysis to make decisions about how to allocate capital across different asset classes. It aims to maximize returns and minimize risk by selecting the most appropriate mix of assets.
Data Science
Data science, on the other hand, has a longer history, with roots in statistics and computer science. Early pioneers such as John Tukey and Claude Shannon laid the foundations for modern data analysis techniques in the 1940s and 1950s. In the early 2000s, the term "data science" started to gain widespread usage, with the launch of the Data Science Journal in 2002 and the establishment of the first data science degree programs in the following years. The field has continued to evolve and grow in popularity in the 21st century, driven by the explosion of data generated by the internet and the proliferation of data-driven technologies such as machine learning and artificial intelligence.
Connections between quant and data science
Today, data science and quantitative investing are closely interconnected, with data scientists playing a key role in the development and implementation of quantitative investment strategies. The combination of data science and quantitative investing allows investors to make more informed and data-driven decisions, leading to improved returns and risk management. Below are ten examples of applying data science in quantitative investing
High-frequency trading: Analyze large amounts of data in real-time to identify trading opportunities and make rapid trades.
Trend following: Identify trends in the market and make investment decisions based on those trends.
Statistical arbitrage: Identify statistical discrepancies in the market and make trades to profit from those discrepancies.
Risk management: Model and analyze risk to identify and mitigate potential losses.
Portfolio construction: Analyze historical data and build portfolios optimized for specific investment objectives.
Factor investing: Identify factors that predict returns, such as value, momentum, and size, and make investment decisions based on those factors.
Sentiment analysis: Analyze social media, news articles, and other sources to gauge investor sentiment and inform investment decisions.
Algorithmic trading: Build algorithms that analyze data and make trades automatically.
Fraud detection: Identify and prevent fraudulent activity in the financial industry.
Predictive modeling: Build models that predict future market movements based on historical data.